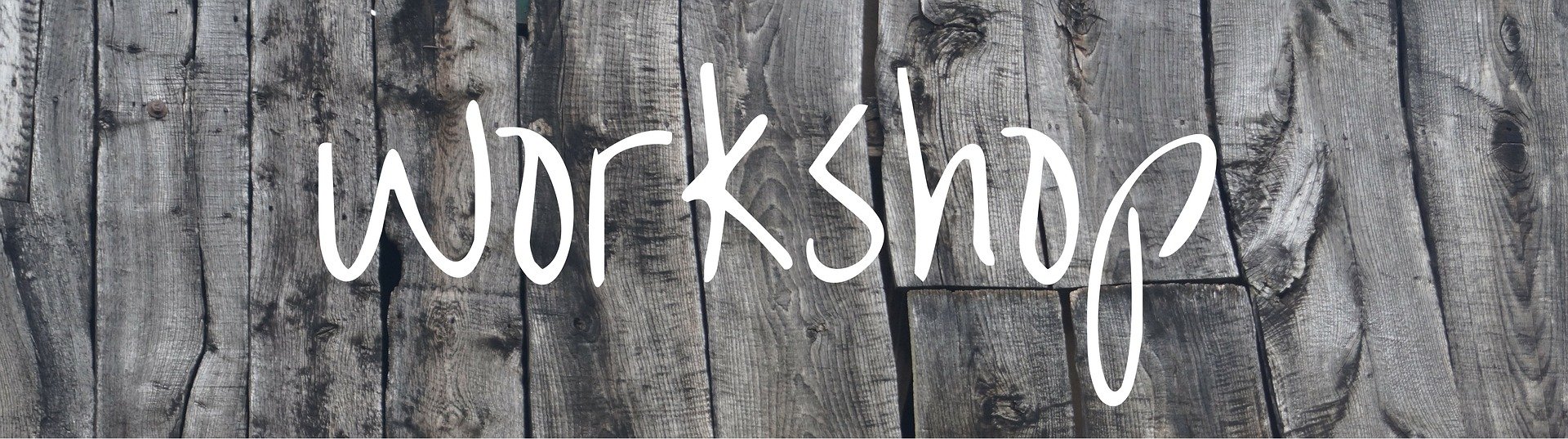
Optimal Control, Optimal Transport and Data Science
IMA – Institute for Mathematics and its Applications with Carnegie Mellon University and IBM organizes the workshop: Optimal Control, Optimal Transport and Data Science
Optimal control and optimal transportation have begun to play an important role in data science. Many techniques of machine learning, including deep learning, high-dimensional statistical learning, transfer learning, anomaly detection, and prediction from expert advice, rely on optimal transport and optimal control to model tasks, compute solutions, and provide theoretical analysis of the algorithms. Optimal transport has been successfully used as a loss function in both theory and practice to provide robust learning approaches. Furthermore, Wasserstein Generative Adversarial Networks (WGAN) have achieved state-of-the-art results for generative learning problems. In deep learning, optimal control of ordinary differential equations has been connected recently with the stability of ResNet neural networks. Reformulation training as an optimal control problem has led to new training algorithms based on Pontryagin’s maximum principle. Furthermore, minimax optimal strategies for minimizing regret have been connected to continuum Hamilton-Jacobi-Isaacs partial differential equations (PDEs). Despite this flurry of work and recent success, many theoretical and computational challenges remain, such as fast computation of optimal transportation distances and maps.
xx