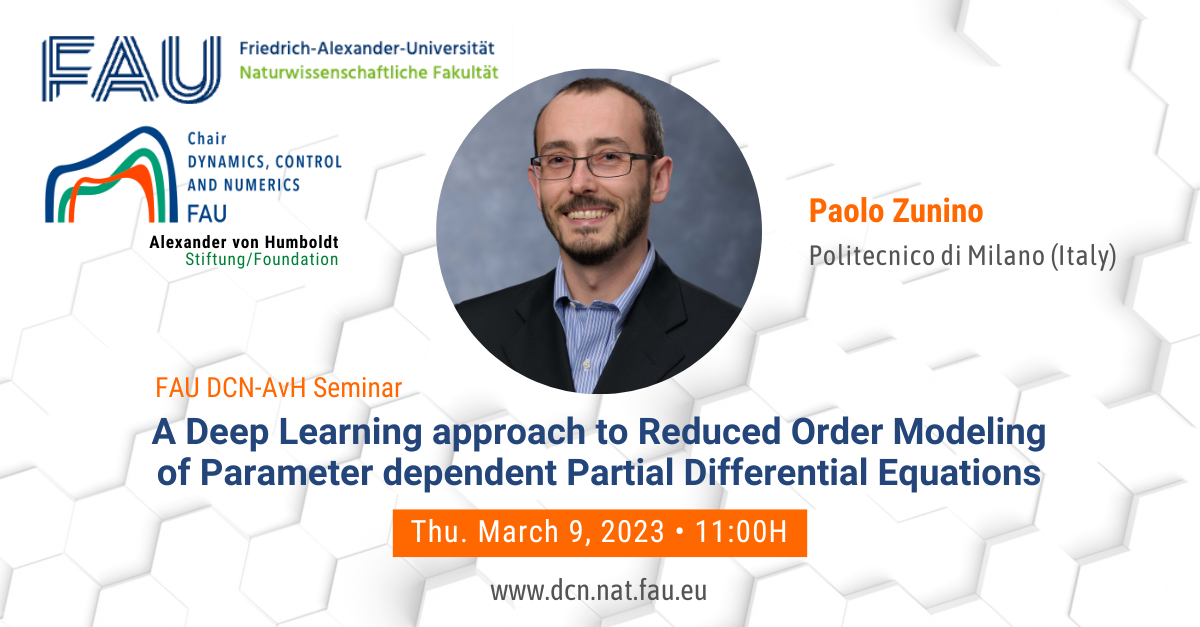
A Deep Learning approach to Reduced Order Modeling of Parameter dependent Partial Differential Equations
Next Thursday March 9, 2023:
Event: FAU DCN-AvH Seminar
Organized by: FAU DCN-AvH, Chair for Dynamics, Control and Numerics – Alexander von Humboldt Professorship at FAU, Friedrich-Alexander-Universität Erlangen-Nürnberg (Germany)
Title: A Deep Learning approach to Reduced Order Modeling of Parameter dependent Partial Differential Equations
Speaker: Prof. Dr. Paolo Zunino
Affiliation: Politecnico di Milano (Italy)
Abstract. In the context of parametrized PDEs, Reduced Order Models (ROMs) allow for an efficient approximation of the parameter-to-solution map, which is extremely useful whenever dealing with expensive many-query routines such as constrained optimization, sensitivity analysis and uncertainty quantification. Recently, many authors have been considering the use of Artificial Neural Networks (ANNs) and Deep Learning techniques for building non-intrusive ROMs.
We discuss a constructive approach based on Deep Neural Networks for the efficient approximation of the parameter-to-solution map of PDEs [1]. Our work is based on the use of deep autoencoders, which we employ for encoding and decoding a high-fidelity approximation of the solution manifold. We show that the minimal latent dimension of the autoencoder is intimately related to the topological properties of the solution manifold, and we provide theoretical results with particular emphasis on second order elliptic PDEs.
We analyze the approximation error of the proposed approach by quantifying how the ROM accuracy scales with respect to the complexity of the networks involved, ultimately providing estimates highlighting the role played by the hyper-parameters defining the architectures. Specific results are also presented for the case of Convolutional Neural networks (CNNs) [2].
The interest in using CNNs is motivated by their ability of learning from complex high dimensional data, while also fostering the trainability of the model, i.e. by yielding architectures that are computationally feasible to optimize; however, their usage is limited to cubic/hypercubic domains. Getting inspiration from CNNs, we propose a new class of architectures, called Mesh-Informed Neural Networks, specifically tailored to handle mesh based functional data, and thus of particular interest for building ROMs of parametrized PDEs [3]. The approach leads to a natural pruning strategy which enables the design of sparse architectures that can learn general nonlinear operators.
The theory presented is further supported by numerical experiments. Applications to the numerical approximation of PDEs with stochastic coefficients and to multiscale problems will be also briefly discussed.
This work is in collaboration with Nicola Rares Franco, Stefania Fresca, Andrea Manzoni, MOX, Department of Mathematics, Politecnico di Milano.
1- Franco, N.R., Manzoni, A., and Zunino P. A deep learning approach to reduced order modelling of parameter dependent partial differential equations. (2023) Mathematics of Computation, 92 (340), pp. 483-524.
2- Franco, N.R., Fresca, S., Manzoni, A., Zunino, P. Approximation bounds for convolutional neural networks in operator learning (2023) Neural Networks, 161, pp. 129-141.
3- Franco, N.R., Manzoni, A., and Zunino, P. Learning Operators with Mesh-Informed Neural Networks (2022) arXiv:2203.11648.
WHERE?
Online:
Zoom meeting link
Meeting ID: 614 4658 159 | PIN code: 914397