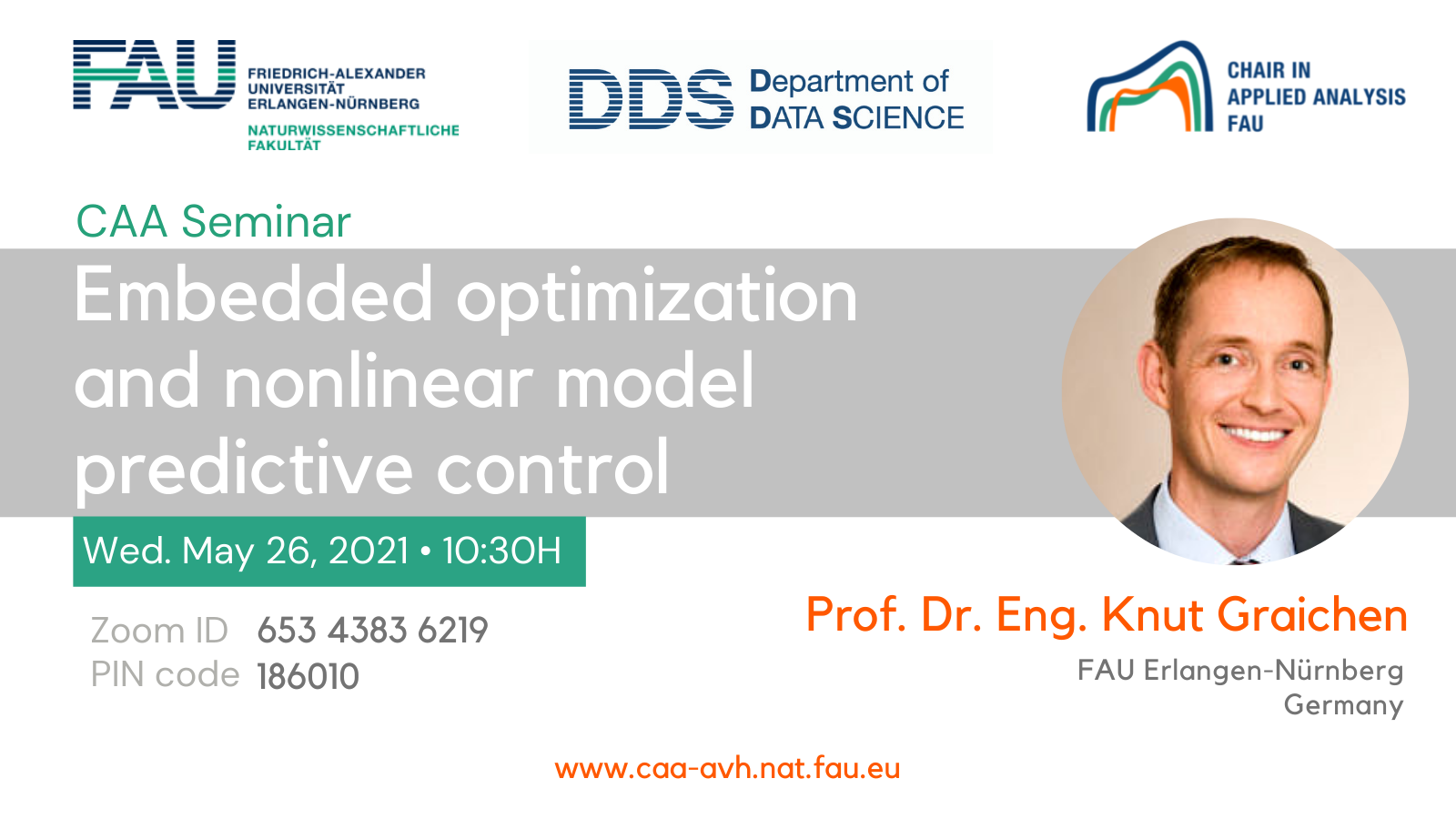
Embedded optimization and nonlinear model predictive control
Speaker: Prof. Dr. Eng. Knut Graichen
Affiliation: FAU Erlangen-Nürnberg, Germany
Organized by: FAU CAA-AvH, Chair in Applied Analysis – Alexander von Humboldt Professorship at FAU Erlangen-Nürnberg (Germany)
Zoom meeting link
Meeting ID: 653 4383 6219 | PIN code: 186010
Abstract. Optimization algorithms have become powerful and established tools for the control and optimization of dynamical systems, e.g. in the context of state estimation, parameter identification and in particular model predictive control (MPC). MPC is an advanced control scheme for linear and nonlinear multiple-input systems that allows to consider system constraints as well as additional control objectives such as energy efficiency. However, a well-known drawback of MPC especially for nonlinear systems is its high computational effort that is related to the online solution of the underlying optimization problem. This challenge is the more severe if mechatronic systems with sampling times in the range of (sub-)milliseconds and weak computational hardware are considered. In order to implement optimization methods and MPC for real-time control purposes therefore requires the design of embedded optimization algorithms with limited complexity while ensuring real-time feasibility and computational efficiency.
The talk addresses the field of embedded real-time optimization and its application to MPC for the control of dynamical systems. It also gives an introduction into the open-source toolbox GRAMPC (“gradient-based augmented Lagrangian framework for embedded NMPC”) developed at the Chair of Automatic Control and demonstrates its performance in comparison with state-of-the-art MPC solvers. Selected mechatronic examples running in the millisecond range are presented and some research extensions in connection with model predictive control are highlighted.