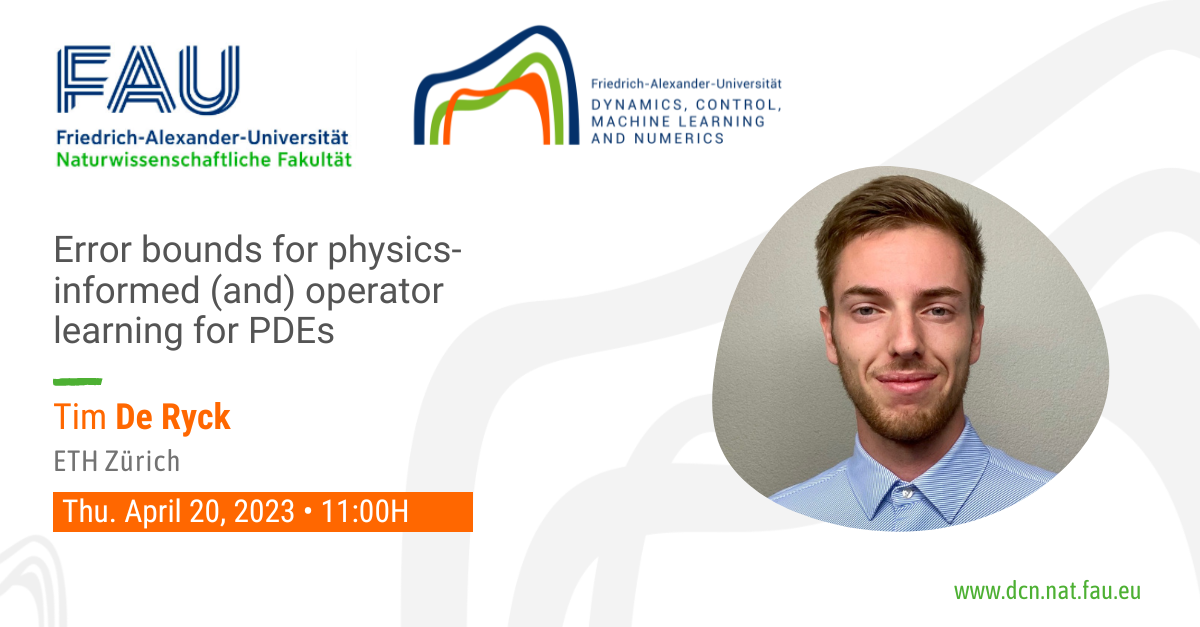
Error bounds for physics-informed (and) operator learning for PDEs
Next Thursday April 20, 2023:
Organized by: FAU DCN-AvH, Chair for Dynamics, Control, Machine Learning and Numerics – Alexander von Humboldt Professorship at FAU, Friedrich-Alexander-Universität Erlangen-Nürnberg (Germany)
Title: Error bounds for physics-informed (and) operator learning for PDEs
Speaker: Tim De Ryck
Affiliation: PhD Student from ETH Zürich
Abstract. In recent years, many deep learning architectures have been proposed to approximate solutions to partial differential equations (PDEs). Despite their impressive performance in experiments, a corresponding mathematical theory is lacking.
We propose a very general framework for deriving rigorous bounds on the approximation error for physics-informed neural networks (PINNs) and operator learning architectures such as DeepONets and FNOs as well as for physics-informed operator learning. These bounds guarantee that PINNs and (physics-informed) DeepONets or FNOs will efficiently approximate the underlying solution or solution operator of generic PDEs. [1]
However, PINNs and related architectures require regularity of the underlying PDE solution to guarantee accurate approximation and may fail at approximating discontinuous solutions of PDEs such as nonlinear hyperbolic equations. To ameliorate this, we propose a novel variant of PINNs, termed as weak PINNs (wPINNs) for accurate approximation of entropy solutions of scalar conservation laws. We prove rigorous bounds on the error incurred by wPINNs and illustrate their performance through numerical experiments to demonstrate that wPINNs can approximate entropy solutions accurately. [2]
Joint work with Prof. Dr. Siddhartha Mishra (ETH Zürich) and Roberto Molinaro (ETH Zürich).
WHERE?
On-site / Online
On-site:
Room Übung 3 | 01.252-128
1st. floor. Department Mathematik. Friedrich-Alexander-Universität Erlangen-Nürnberg
Cauerstraße 11, 91058 Erlangen
GPS-Koord. Raum: 49.573572N, 11.030394E
Online:
Zoom meeting link
Meeting ID: 614 4658 159 | PIN code: 914397