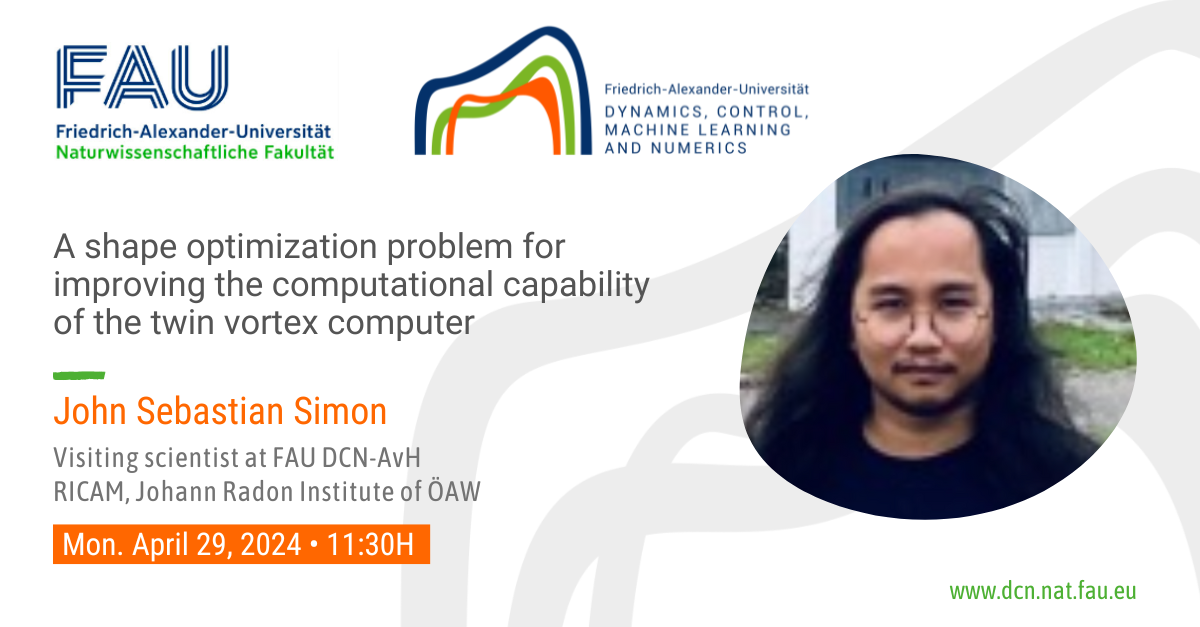
A shape optimization problem for improving the computational capability of the twin vortex computer
Next Monday April 29, 2024:
Organized by: FAU DCN-AvH, Chair for Dynamics, Control, Machine Learning and Numerics – Alexander von Humboldt Professorship at FAU, Friedrich-Alexander-Universität Erlangen-Nürnberg (Germany)
Title: A shape optimization problem for improving the computational capability of the twin vortex computer
Speaker: John Sebastian Simon
Affiliation: Johann Radon Institute for Computation and Applied Mathematics (RICAM), Austria
Abstract. Physical reservoir computing is a new computational paradigm based on recurrent neural networks where instead of optimizing nodal connection of the internal network, one gets to utilize the nonlinear behavior of physical systems and gets to focus on optimizing a minimal number of parameters. In 2021, Goto et al [1] investigated a physical reservoir computer in the context of a flow past a cylinder and shown that the computer’s computational capability is maximized at the bifurcation point between the generation of twin vortex and the onset of Karman vortex.
In this exposition, the authors illustrated how the dynamics of twin vortex affects the ability of the computer to accomplish certain tasks. In particular, they have shown that the length of the twin vortex is directly proportional to the performance.
In this talk, a shape optimization problem that aims to increase the said capability will be presented. The optiimization problem is a naive formulation by maximizing two types of functional which has been historically used a quantifiers of vortex, namely, the L2-norm of the curl of the velocity and the positivity of the determinant of the velocity gradient. The optimization problem is regularized by a perimeter functional which acts as a Tikhonov regularizer. A volume constraint is also imposed, which — together with the regularizer — prevents possible topological changes in the domain. The analysis of this problem includes existence analysis of the governing state, establishing the existence of shape solutions, and sensitivity of the objective functional with respect to domain perturbation. We shall then utilize the results of the sensitivity analysis to a gradient descent-type algorithm for numerical illustrations.
[1] K. Goto, K. Nakajima, and H. Notsu, Twin vortex computer in fluid flow, New J. Phys., 23 (2021), p. 063051, https://doi.org/doi.org/10.1088/1367-2630/ac024d.WHEN
Mon. April 29, 2024 at 11:30H
WHERE
On-site: Room 03.323
Friedrich-Alexander-Universität Erlangen-Nürnberg
Cauerstraße 11, 91058 Erlangen
GPS-Koord. Raum: 49.573764N, 11.030028E
_
See all Seminars at FAU DCN-AvH
Don’t miss out our last news and connect with us!
LinkedIn | Twitter | Instagram
Speaker
-
John Sebastian SimonRICAM