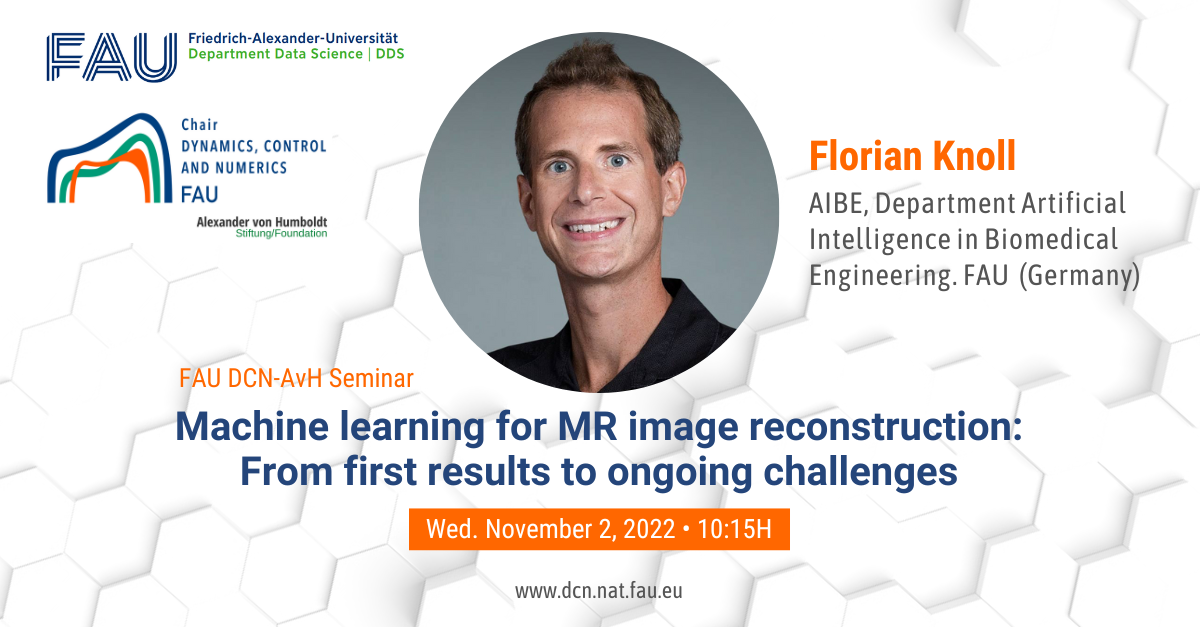
Machine learning for MR image reconstruction: From first results to ongoing challenges
Next Wednesday, November 2, 2022:
Event: FAU DCN-AvH Seminar
Organized by: FAU DCN-AvH, Chair for Dynamics, Control and Numerics – Alexander von Humboldt Professorship at FAU, Friedrich-Alexander-Universität Erlangen-Nürnberg (Germany)
Title: TBA
Speaker: Prof. Dr. Florian Knoll
Affiliation: AIBE, Department Artificial Intelligence in Biomedical Engineering. FAU Friedrich-Alexander-Universität Erlangen-Nürnberg (Germany)
WHERE?
On-site / online
On-site:
Room 04.363
Friedrich-Alexander-Universität Erlangen-Nürnberg
Department Informatik (next to Übungraum 01.254 of Department Mathematik.)
Cauerstraße 11, 91058 Erlangen
GPS-Koord. Raum: 49.573713N, 11.030428E
Online:
Zoom meeting link
Meeting ID: 614 4658 159 | PIN code: 914397
Abstract. Six years ago, machine learning techniques have been first introduced to solve the inverse problem of MR image reconstruction from undersampled data from accelerated acquisitions (1,2,3). Since then, the field has grown substantially, and a wide range of machine learning methods have been developed and applied to a wide range of imaging applications. In this talk, I will start with the background of a machine learning reconstruction that is based on iterative reconstruction methods used in compressed sensing and maps the reconstruction problem onto a neural network. I will discuss advantages and ongoing challenges, covering design of the network architecture and the training procedure, error metrics, computation time, generalizability and validation of the results. This includes a discussion of the lessons learnt from the recent fastMRI image reconstruction challenges (4,5).
References
1. Learning a variational model for compressed sensing MRI reconstruction. Hammernik, et al. Proc. ISMRM p33 (2016).
2. Accelerating magnetic resonance imaging via deep learning. Wang et al. IEEE ISBI 514-517 (2016).
3. Hammernik et al. Learning a Variational Network for Reconstruction of Accelerated MRI Data. MRM, 79:3055-3071 (2018).
4. Advancing machine learning for MR image reconstruction with an open competition: Overview of the 2019 fastMRI challenge. Knoll et al. MRM 84 (6), 3054-3070 (2020).
5. Results of the 2020 fastmri challenge for machine learning MR image reconstruction. Muckley et al. IEEE TMI 40 (9), 2306-2317(2021).