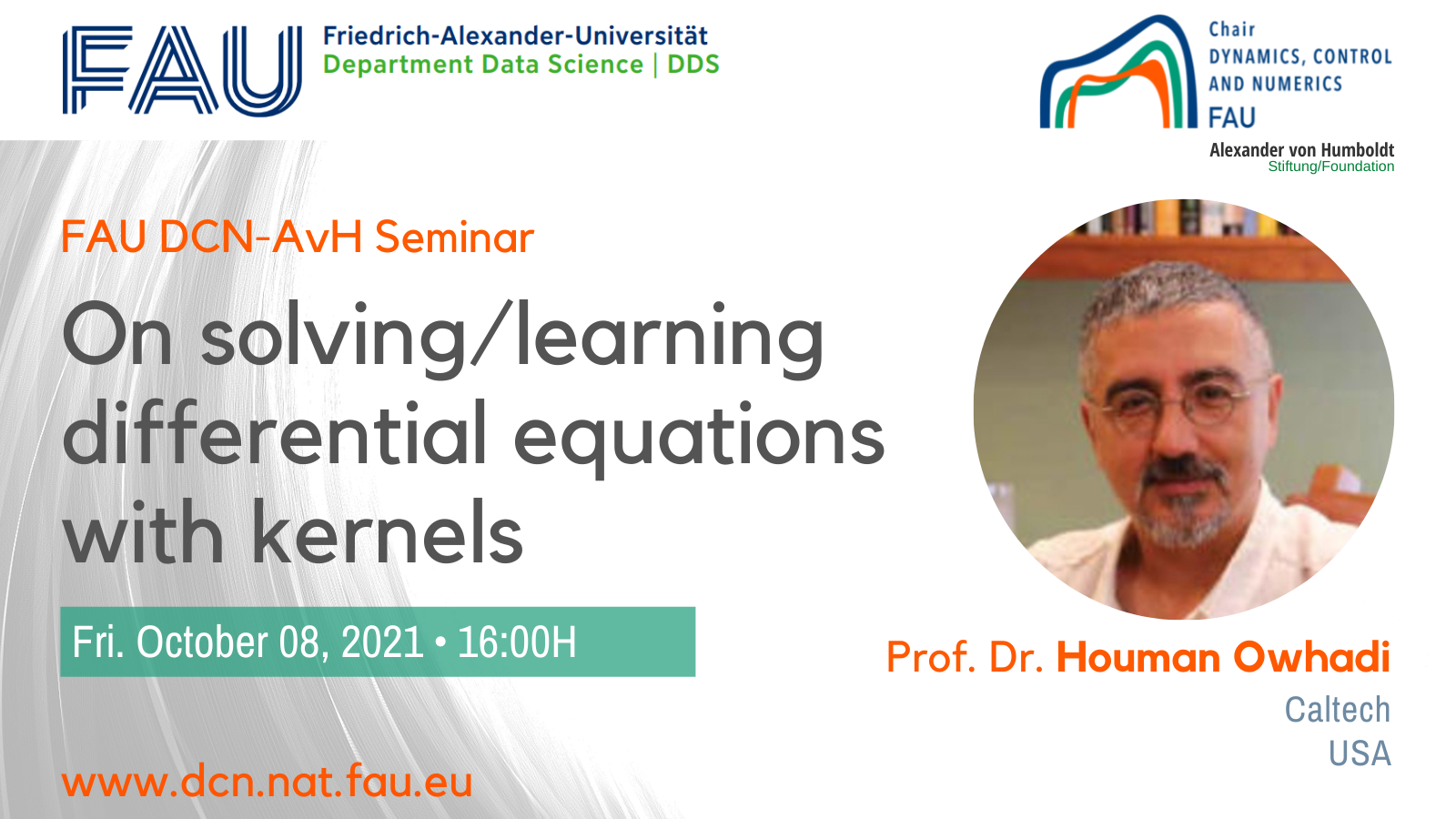
On solving/learning differential equations with kernels
Speaker: Prof. Dr. Houman Owhadi
Affiliation: Caltech (USA)
Organized by: FAU DCN-AvH, Chair for Dynamics, Control and Numerics – Alexander von Humboldt Professorship at FAU Erlangen-Nürnberg (Germany)
Zoom meeting link
Meeting ID: 686 4453 8133 | PIN code: 603054
Abstract. We present a simple, rigorous, and unified framework for solving and learning (possibly nonlinear) differential equations (PDEs and ODEs) using the framework of Gaussian processes/kernel methods.
For PDEs the proposed approach: (1) provides a natural generalization of collocation kernel methods to nonlinear PDEs and Inverse Problems; (2) has guaranteed convergence for a very general class of PDEs, and comes equipped with a path to compute error bounds for specific PDE approximations; (3) inherits the state-of-the-art computational complexity of linear solvers for dense kernel matrices. For ODEs, we illustrate the efficacy of the proposed approach by extrapolating weather/climate time series obtained from satellite data and highlight the importance of using adapted/learned kernels.
Parts of this talk are joint work with Yifan Chen, Boumediene Hamzi, Bamdad Hosseini, Romit Maulik, Florian Schäfer, Clint Scovel and Andrew Stuart.